Almost 85% of the 1.7 million youth living with HIV are situated in sub-Saharan Africa, alongside half of the approximately 40 million individuals globally infected with HIV. While the Ugandan government offers complimentary antiretroviral therapy (ART), the compliance to the treatment protocol among adolescents aged 10-16 is minimal, heightening the risk of further transmission of the virus.
Claire Najjuuko, a PhD candidate at Washington University in St. Louis, observed this situation up close during her tenure as a data administrator at the International Center for Child Health and Development (ICHAD) in Uganda, established by Fred M. Ssewamala, the William E. Gordon Distinguished Professor at the WashU Brown School. Currently pursuing a doctorate in WashU’s Division of Computational & Data Sciences, Najjuuko aims to leverage artificial intelligence (AI) and data science to enhance adolescent adherence to treatment in resource-limited regions. Her co-mentors include Ssewamala and Chenyang Lu, the Fullgraf Professor of computer science and engineering at the McKelvey School of Engineering. The findings of their research were published online on Feb. 25 in the journal AIDS.
“I am deeply passionate about machine learning and aspire to apply it to issues that resonate with me,” she remarked. “The partnerships between the AI for Health Institute, led by Professor Lu, and the International Center for Child Health and Development, directed by Professor Fred, significantly facilitate this type of innovative endeavor.”
With the backing of Lu and Ssewamala, Najjuuko endeavored to create a machine-learning model to predict which adolescents with HIV are less likely to remain compliant with antiretroviral therapy. By understanding which individuals may struggle with adherence, healthcare professionals can target interventions for those deemed less likely to follow treatment guidelines.
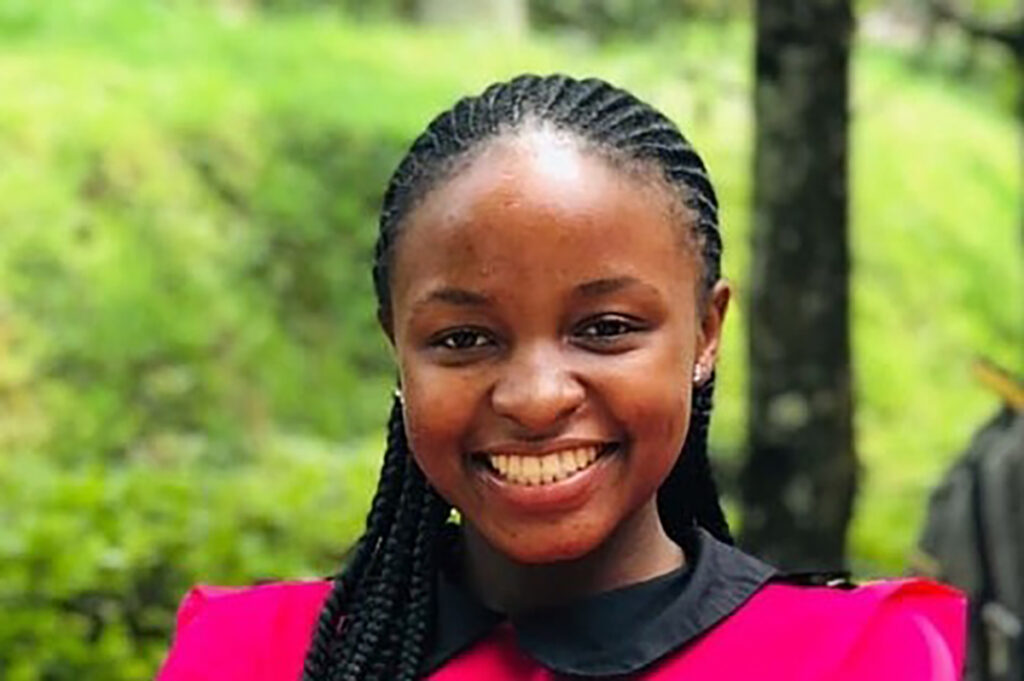
“The existing procedure involves adolescents visiting the clinic every month or two months for prescription renewals, where a healthcare provider checks how many pills remain relative to what is anticipated and questions the adolescent about missed doses to determine if the patient is following the treatment regimen,” Najjuuko explained. “This initiative aimed at predicting future nonadherence among adolescents could create a substantial impact if carried out correctly.”
To train the model, Najjuuko utilized data from a six-year cluster-randomized controlled trial performed across 39 clinics in southern Uganda, a region severely affected by HIV. The Suubi+Adherence dataset comprised adolescents aged 10-16 who were medically diagnosed with HIV; were aware of their condition; enrolled in ART at a participating clinic; and resided in a family setting. Ultimately, the models evaluated data from 647 patients who had complete information on the outcome after 48 months.
Najjuuko constructed a machine-learning model to forecast nonadherence to antiretroviral therapy by integrating sociobehavioral and economic factors along with a patient’s adherence history. The model successfully identifies 80% of adolescents at risk of nonadherence while decreasing the false alarm rate to 52% — a 14 percentage point improvement compared to a model reliant solely on adherence history. By minimizing false alarms, this model enables healthcare providers to concentrate interventions on those who require them the most, enhancing patient outcomes while curtailing unnecessary follow-ups and provider exhaustion.
Among 50 variables, encompassing social, interpersonal, family, educational, structural, and economic factors, the model identified 12 as the most indicative of poor adherence to ART. Economic factors showed robust associations with future nonadherence. Other indicative traits included a poor adherence history; childhood poverty; biological connections to the primary caregiver; self-perception; confidence in saving; discussing sensitive issues with caregivers; household size; and school enrollment.
“Adolescents represent the most noncompliant demographic worldwide,” stated Ssewamala. “They are transitioning into independence and resist being directed in their actions. When they enter the dating phase, stigma becomes prevalent, and they avoid association with HIV.”
One aspect the team discovered that correlated with adolescents adhering to ART was the possession of a savings account.
“The hypothesis is that when individuals own resources, especially having a financial cushion, their mindset and behaviors change,” Ssewamala explained. “Hope for the future motivates them to care for themselves to enhance their longevity. Conversely, when individuals feel hopeless, they perceive they have nothing to lose.”
Adherence to treatment poses challenges, Ssewamala added, as the medication must be taken with food or it induces nausea. If an individual with HIV lacks access to food or means to procure their medication, their likelihood of adhering to the treatment decreases.
Lu remarked that this model could be fine-tuned for real-world application to support personalized intervention approaches grounded in the identified risk factors, underscoring the significance of collaboration.
“This exemplifies exceptional interdisciplinary research at WashU, merging AI with global health,” Lu stated. “By leveraging the data that Fred’s team collected on the ground and their comprehension of intricate health challenges, we utilize AI expertise to evaluate this data and develop tools that improve health outcomes.”
Najjuuko C, Brathwaite R, Xu Z, Kizito S, Lu C, Ssewamala FM. Utilizing machine learning to forecast poor adherence to antiretroviral therapy among adolescents living with HIV in under-resourced settings. AIDS. Online Feb. 25, 2025, DOI:10.1097/QAD.0000000000004163.
Funding for this study was provided by the Eunice Kennedy Shriver National Institutes of Child Health and Human Development (NICHD) (1RO1HD074949-01).
Initially published on the McKelvey Engineering website
The article Machine learning could help predict adherence to HIV treatment was first featured on The Source.