Chemists from Florida State University have developed a machine learning tool capable of determining the chemical makeup of dried salt solutions from an image with 99% precision.
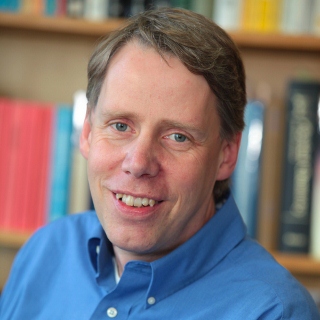
By employing robotics to generate thousands of samples and artificial intelligence to interpret the subsequent data, they fashioned a straightforward, low-cost instrument that could broaden the potential for conducting chemical analyses. This research was published in Digital Discovery.
“We are currently in the era of artificial intelligence and extensive data,” stated co-author Oliver Steinbock, a professor in the FSU Department of Chemistry and Biochemistry. “We believed that by utilizing sufficiently extensive databases containing numerous images of various compounds and stains, AI might help us ascertain the chemical composition.”
This study has the potential to lead to more affordable, quicker chemical assessments applicable in fields such as space exploration, law enforcement, home testing, and beyond.
HOW IT FUNCTIONS
This manuscript builds upon an earlier investigation from Steinbock’s laboratory, where researchers utilized machine learning to discern the chemical makeup of salt stains captured in photographs. In that investigation, approximately 7,500 samples were manually prepared and analyzed by the researchers.
The current paper enhances that study by using a robotic system to process samples, which were subsequently examined with an advanced machine learning program. Instead of manually pipetting samples, the researchers devised a system dubbed the Robotic Drop Imager, or RODI, which is capable of preparing over 2,000 samples daily. This development enabled them to compile a collection of more than 23,000 images — more than threefold compared to their initial research.
Upon preparing samples and capturing images, the researchers streamlined each picture by converting them to grayscale, extracting 47 distinct features such as pattern area, brightness, and other characteristics for their analysis.
With the inclusion of additional images, the accuracy of their machine learning program surged from approximately 90% to nearly 99%. The researchers also examined the initial concentration of the salt solution at five distinct levels, training their machine learning program to differentiate among them. The program achieved 92% accuracy in identifying both the concentration of the solution and the identity of the salt.
“The precision required for various analyses will differ based on the circumstances,” remarked co-author Amrutha S.V., a postdoctoral researcher. “From my experience, I am aware that some forms of spectroscopy and other analytical techniques are costly and necessitate specialized technical knowledge to operate. This is why I’m thrilled about the prospect of a simple method — merely taking a photograph to ascertain chemical composition. That would be immensely beneficial.”
The article ‘Democratizing chemical analysis’: FSU chemists utilize machine learning and robotics to recognize chemical compositions from images first appeared on Florida State University News.