“`html
Health
Detecting brain cancer in children before its recurrence
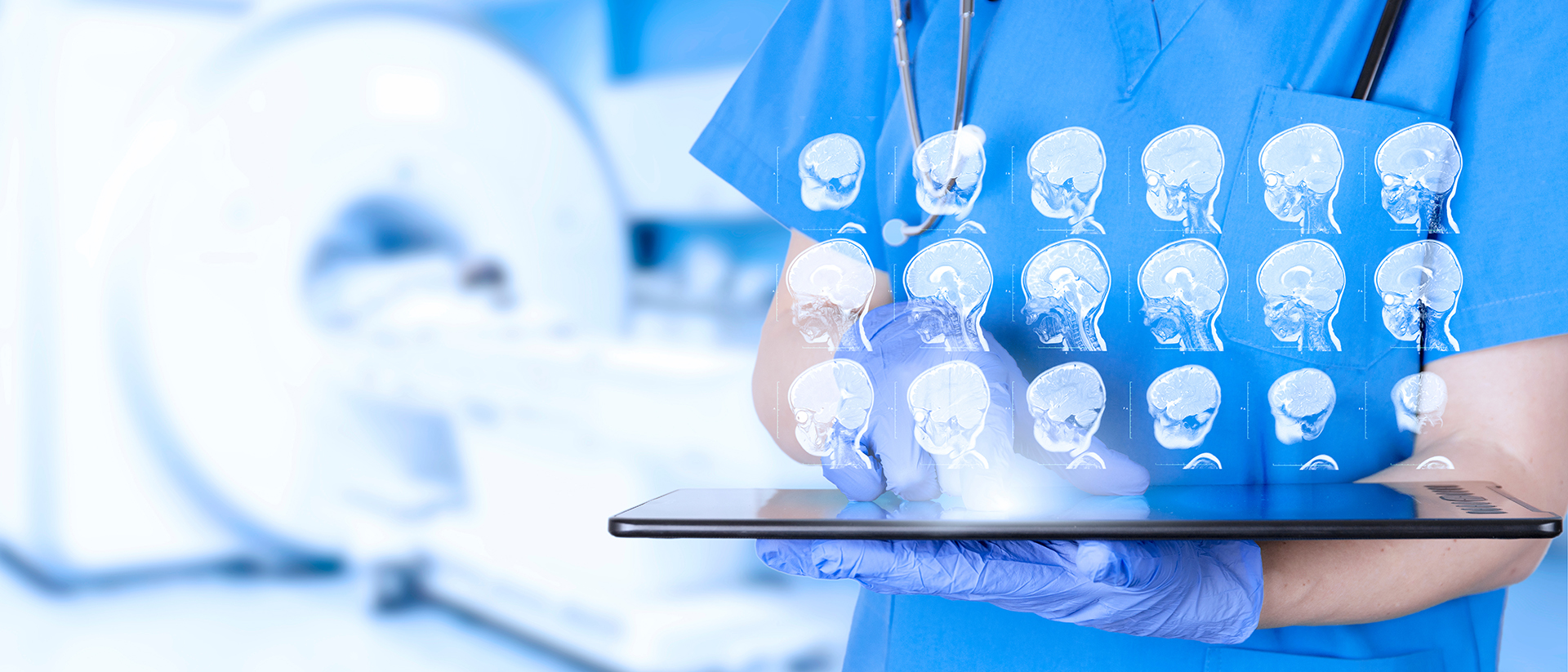
AI tool outperforms traditional methods in predicting relapse risk in Harvard study
An AI system designed to assess various brain scans over time forecasted relapse risk in pediatric cancer patients with significantly higher accuracy than conventional methods, as per a recent study. Researchers are optimistic that these findings will enhance treatment for children afflicted with brain tumors known as gliomas, which are usually treatable but differ in their likelihood of recurrence.
“Numerous pediatric gliomas can be cured solely through surgery, but when they recur, the consequences can be severe,” stated corresponding author Benjamin Kann from the Artificial Intelligence in Medicine Program at Mass General Brigham and the Department of Radiation Oncology at Brigham and Women’s Hospital. “It is extremely challenging to identify individuals who may be at risk of recurrence, resulting in patients undergoing frequent follow-ups with magnetic resonance imaging for several years, a procedure that can be taxing and distressing for both children and families. We require more effective tools to pinpoint early which patients are at the greatest risk of recurrence.” Kann also serves as Assistant Professor of Radiation Oncology at Harvard Medical School.
The findings of the study conducted by researchers from Mass General Brigham and partners at Boston Children’s Hospital and Dana-Farber/Boston Children’s Cancer and Blood Disorders Center were published in The New England Journal of Medicine AI.
The research, partially funded by the National Institutes of Health, utilized institutional collaborations nationwide to gather nearly 4,000 MR scans from 715 pediatric patients. To optimize what AI could “comprehend” from a patient’s brain scans — and to forecast recurrence more precisely — the researchers utilized a methodology known as temporal learning, training the model to compile information from numerous brain scans taken over several months following surgery.
“Numerous pediatric gliomas can be cured solely through surgery, but when they recur, the consequences can be severe.”
Benjamin Kann
Typically, AI models in medical imaging are trained to derive insights from individual scans; with temporal learning, a method not previously applied in medical imaging AI research, images collected over time enhance the algorithm’s forecast of cancer recurrence. To create the temporal learning model, the researchers initially trained it to arrange a patient’s post-surgery MR scans in chronological sequence, enabling it to recognize subtle modifications. Subsequently, the researchers refined the model to accurately connect changes with ensuing cancer recurrence, when relevant.
Ultimately, the researchers discovered that the temporal learning model predicted the recurrence of either low- or high-grade gliomas one year post-treatment, achieving an accuracy rate of 75-89 percent — significantly higher than the accuracy linked to predictions based on single images, which they found to be approximately 50 percent (no better than random chance). Providing the AI with more images over various time points post-treatment improved the model’s predictive accuracy, yet only four to six images were necessary before this enhancement plateaued.
The researchers advise that additional validation in further contexts is essential before clinical implementation. Ultimately, their goal is to initiate clinical trials to ascertain whether AI-informed risk predictions can lead to advancements in care — either by diminishing imaging frequency for lower-risk patients or by proactively treating high-risk patients with targeted adjuvant therapies.
“We have demonstrated that AI can effectively analyze and predict from multiple images, not just individual scans,” remarked first author Divyanshu Tak from the AIM Program at Mass General Brigham and the Department of Radiation Oncology at Brigham. “This method may be applicable in many situations where patients receive serial, longitudinal imaging, and we’re eager to see what this project will inspire.”
This study was partially supported by the National Institutes of Health/the National Cancer Institute.
“`